LLM vs Generative AI: A Simple Guide to All You Need to Know
We’ve seen an explosion in AI in the last few years. So come along as we explore and compare two AI models that are reshaping our digital landscape and our daily lives – LLM vs Generative AI.
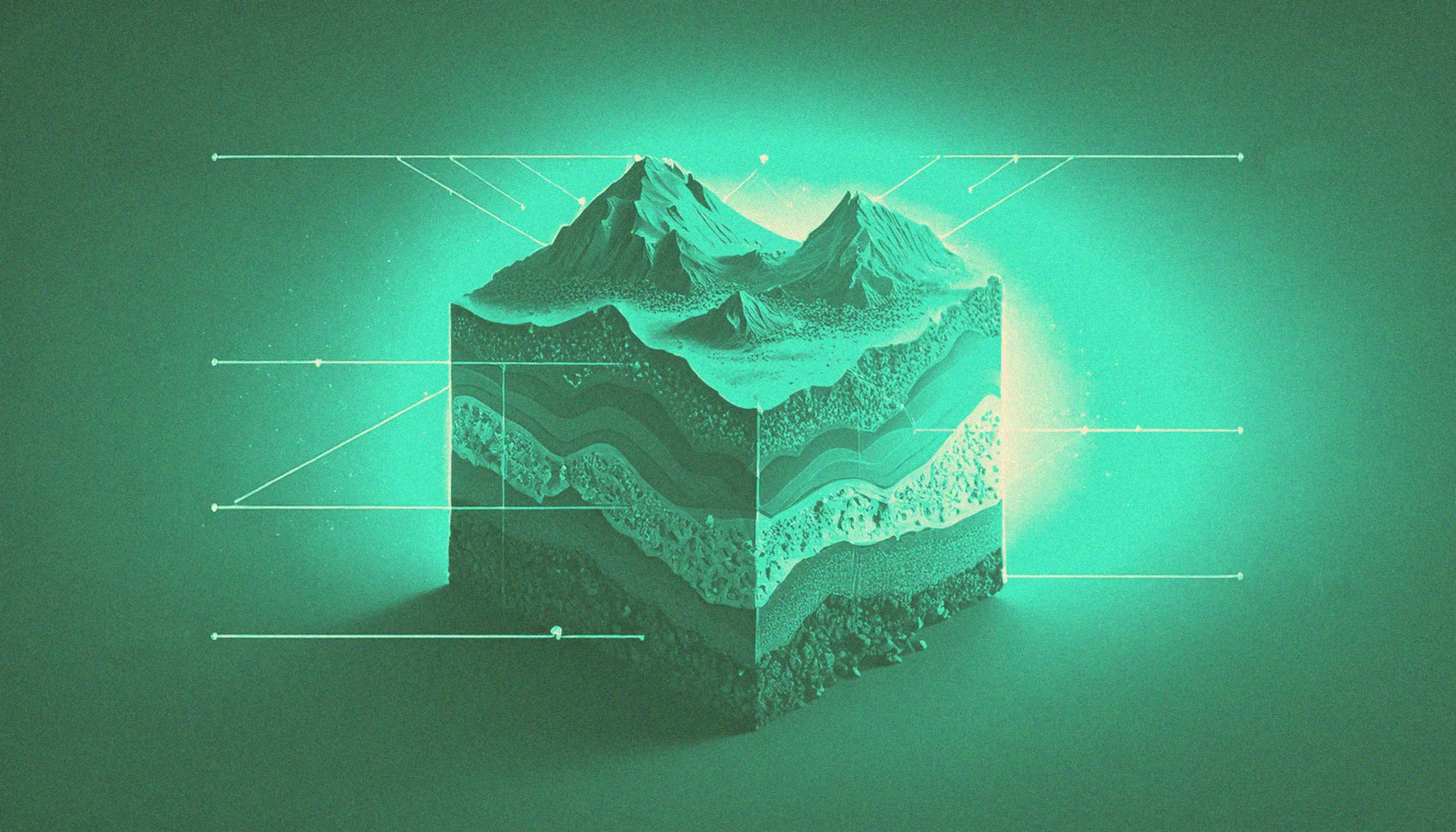
The rise of AI-based tools is responding to an increasing gap, forcing industries and businesses to rapidly adapt and evolve. This space, which we can call generative artificial intelligence, is attracting more and more sectors by the day and pushing them toward change. A common question arises for users and business leaders alike as they start to experiment with different models: LLM vs Generative AI – what are the key differences?
What Is Generative AI?
Generative artificial intelligence is a big bag that holds all types of AI that create different kinds of content, from chatbots such as ChatGPT to image tools like Adobe Firefly. Generative AI learns the structure of the input data and then generates output data with similar characteristics.
There’s this one sentence that’s easy to remember when talking about GenAI:
“Not all GenAI tools are LLMs, but all LLMs are a form of GenAI.”
A packed bubble chart visualizing different AI models.
What is a Large Language Model (LLM)?
A large language model is a deep learning model that is pre-trained on a huge amount of data. It’s also a specific kind of generative AI focused on natural language processing tasks such as understanding and generating text. An LLM can write an essay, summarize a book, or answer your profoundly thoughtful questions.
Regarding LLMs, there’s one thing you ought to remember:
“LLMs’ capabilities stem from extensive training of vast amounts of data. Still they do not possess the true understanding of their responses, as their outputs are based on patterns in the data they were trained on. “
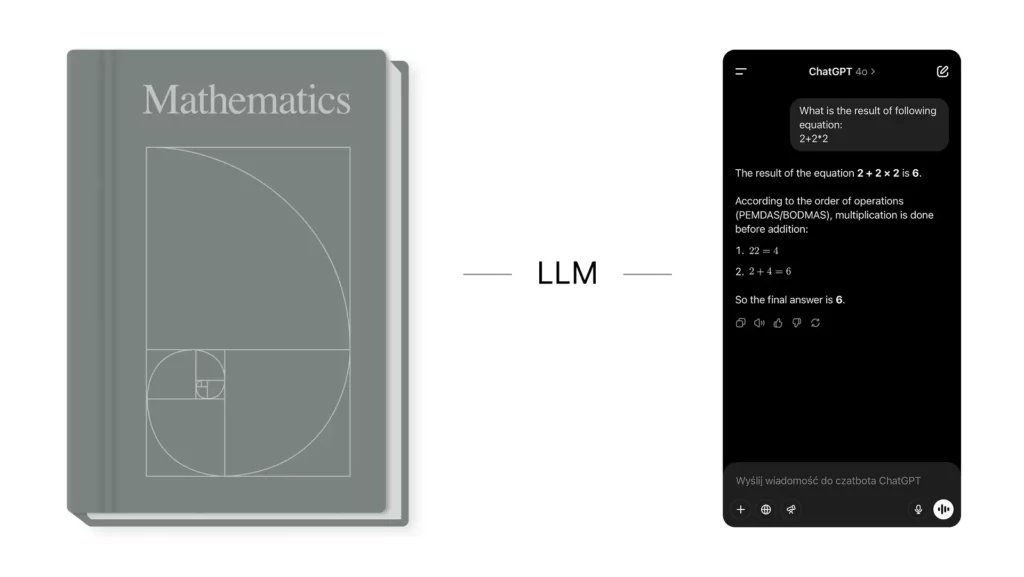
Definitions and theory are great, but as Linus Torvalds (Creator of the Linux kernel) once said “Theory and practice sometimes clash. And when that happens, theory loses. Every single time.”
Will theory lose in combat with practice this time too? Let’s find out by looking at real-life examples of LLMs and generative AI tools.
Examples of LLM & GenAI Tools
Nowadays, with AI being a buzzword, there are dozens of LLMs and generative AI tools, but here we’ve picked the most popular ones you’ve probably heard of.
Generative AI Examples
The tool is specifically designed for visual content creation rather than text generation. It’s also trained on visual data, like Adobe Stock imagery or openly licensed visual content, not on giant containers of text, making it a great example of a generative AI tool.
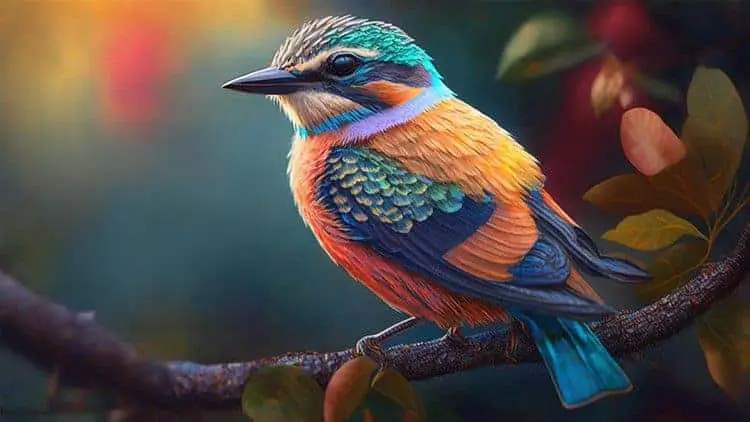
Like in the previous example, DeepDream is another tool for creating visuals. Yes, it uses text prompts (btw like Adobe Firefly) as input, but it doesn’t generate or process complex text like an LLM would. Instead it interprets these prompts to create visual outputs.
Curiosity: DeepDream transforms ordinary images into surreal, psychedelic visions. It utilizes neural networks to analyze and enhance patterns it “sees” in photos – which is why transformed images often feature eyes, animal faces, or wavy structures. The effect resembles dreams or hallucinations, and the longer the algorithm works on an image, the more abstract it becomes. DeepDream has found applications in generative art, AI exploration, and visual effects, offering a fascinating glimpse into how artificial intelligence “interprets” reality.
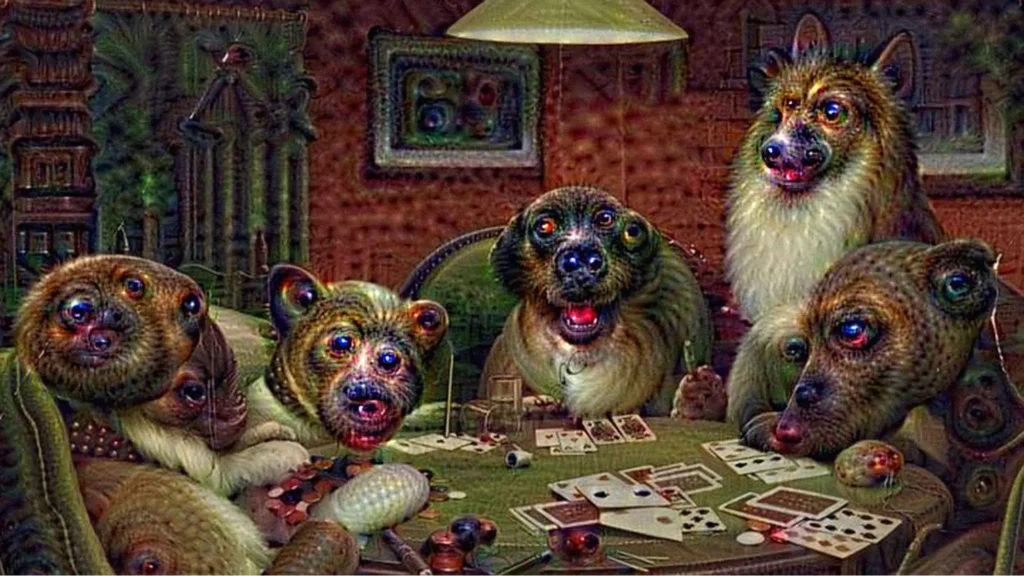
Midjourney is a generative AI tool that creates images based on textual descriptions. It works through Discord, where users enter commands and the algorithm generates unique graphics. It is utilized by artists, designers, and marketers, among others, bridging the gap between art and technology and redefining the creative process.
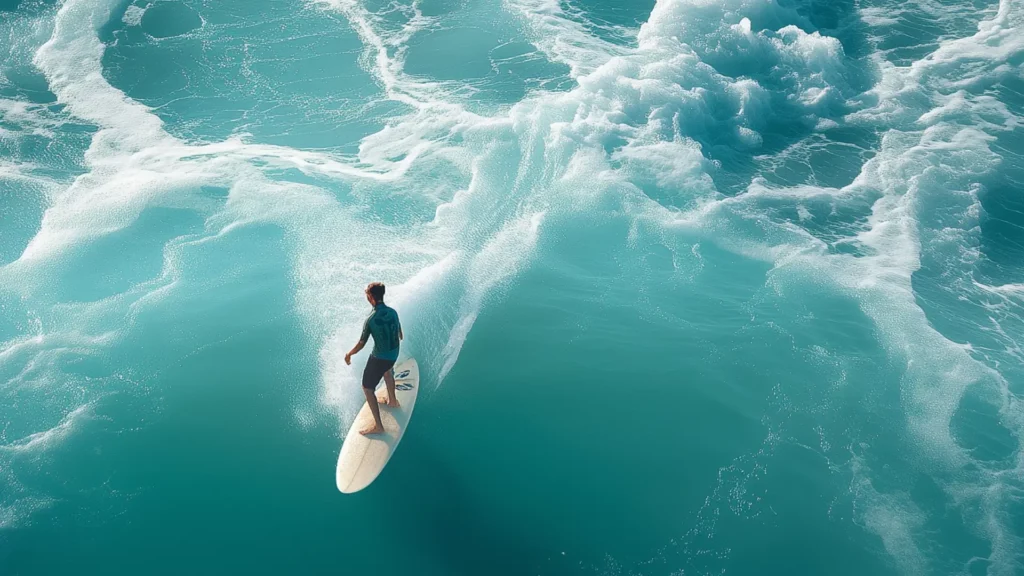
LLM AI Examples
One of the most advanced and widely-discussed LLMs. GPT-4 can handle both text and image inputs, making it a multimodal model.
Is GPT-4 an LLM as it can also process images?
This is a nuanced topic, as LLms are limited to processing text right? The thing is that huge LLMs have evolved over time to also process other types of content. This makes them a multimodal model, not a specific one focused on images or music, for example.
The key is to understand the specific capabilities of each model rather than relying solely on the terminology.
Known for its strong performance across a wide range of tasks, from coding to creative writing. The model’s exact size and architecture are not publicly disclosed, but it’s believed to have hundreds of billions of parameters.
To show how impressive the level of sophistication of the GPT Chat is, we asked it to show for help a generated photo showing its development from a classic LLM to a multimodal model. Here’s the artwork it returned. Isn’t this proof enough that artificial intelligence is thinking more and more abstractly?
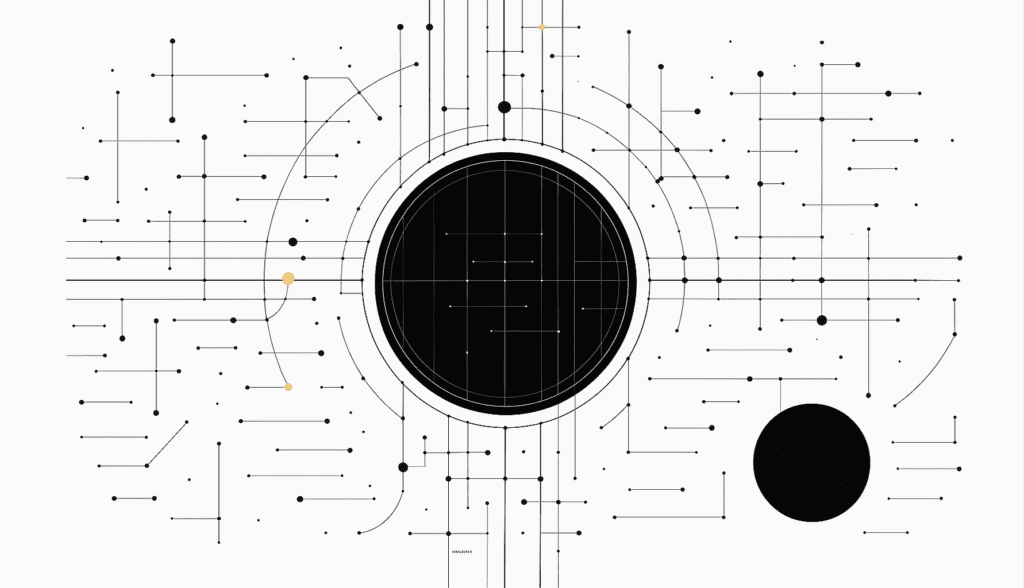
The biggest competitor to GPT-4. It’s also a multimodal model that can process and generate text, images, audio, and video. As Gemini is Google’s AI model it’s also integrated into Google’s AI chatbot and various Google services, enhancing their capabilities.
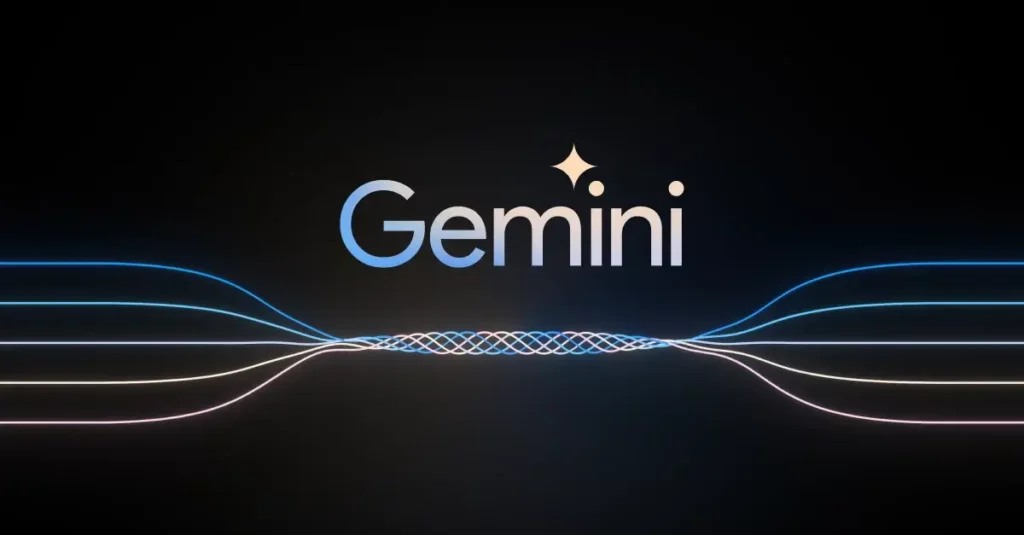
Practice and theory. I’m afraid Linus was right, and theory lost this combat. Especially when we’re talking about multimodal models, the definitions become quite unclear. Still, a table of differences between our main heroes that might be handy for you.
LLM vs Generative AI – Key Differences
When comparing Large Language Models (LLMs) and Generative AI (GenAI), it’s important to consider their outputs, strengths, and potential risks. These differences help users choose the right tool while also highlighting possible concerns.
Here you can take a look at those differences:
Large Language Model (LLM) | Generative AI (GenAI) | |
Outputs | Generates mainly text-based content, such as articles, summaries, and conversations. | Produces various types of content, including text, images, videos, music, and even 3D models. |
Strengths | Advanced language comprehension, capable of generating text at scale. Used in chatbots, search engines, and automated content writing. | Mimics human creativity, generating images, music, and video. Applied in digital art, film production, and game design. |
Concerns | Risk of misinformation due to AI hallucinations, potential for political propaganda, and the generation of biased or misleading content. | Ethical issues, deepfakes, bias, and copyright infringement. GenAI can produce fake media, increasing risks related to misinformation. |
Going Beyond the Division – Multimodal Models
LLMs, which initially operated only on text, have gained a new dimension through multimodality. Integration with models that process images, sound, or video allows them not only to understand text but also to analyze and generate diverse content. By combining with computer vision models, as seen in GPT-4V or Gemini, AI can describe images, interpret charts, or even recognize emotions on faces. Multimodality also paves the way for more interactive communication—systems can translate speech in real-time or even generate videos based on text, like OpenAI’s Sora.
The fusion of different modalities makes AI more intuitive and brings it closer to human-like information processing. LLMs can now not only answer questions but also “see” and “hear,” enabling them to tackle more complex tasks. This is a crucial step toward creating truly versatile artificial intelligence that integrates language, vision, and sound into a single model. People are already applying these capabilities in medicine, education, and even creative arts. The future of AI will be based on models that can seamlessly work across different data formats, making interactions with them even more natural.
Conclusion: The Future of AI – A Synergy of Generative AI and LLMs
As artificial intelligence continues to evolve, the distinction between Generative AI and Large Language Models (LLMs) becomes more than just a technical comparison—it highlights the growing complexity and versatility of AI. While LLMs excel in understanding and generating text, Generative AI expands creative possibilities by producing visual, auditory, and multimedia content.
Rather than competing, these models complement each other, paving the way for a new generation of AI-powered tools that blend language comprehension with creative expression. From revolutionizing content creation to enhancing business automation, both technologies play a vital role in shaping the digital landscape.
However, as their capabilities grow, so do ethical considerations. Misinformation, bias, and the authenticity of AI-generated content remain critical challenges that developers and policymakers must address. The key to responsible Artificial Intelligence adoption lies in transparency, regulation, and continuous improvement.
Ultimately, the future of AI is not about choosing between Generative AI or LLMs, but about harnessing their combined power to create smarter, more innovative, and ethically responsible AI solutions.
Artificial intelligence is a fantastic tool with virtually unlimited potential. The past few years have seen exponential technological growth. If you’d like us to guide you through the world of AI solutions in data visualization, feel free to contact us.
At Black Label, we build data-rich interfaces and applications that help people make better decisions.
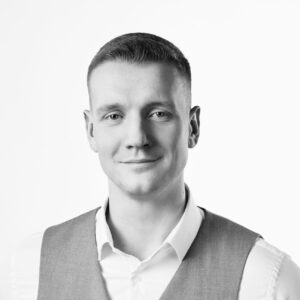